It’s no surprise that marketers, founders, and business owners are starting to ask how their brands can be returned in the answers given by AI/LLM tools like ChatGPT, Perplexity, and Gemini. But it’s downright shocking how difficult it is to find high-quality, accurate information about how to do this.
Granted, you could just ask ChatGPT (the first answer is decent, as are suggestions #2-10 to the second question; #1 is… problematic, more about that in the video). Getting to the heart of the matter, however, requires a solid understanding of how and why LLMs return the answers they do. Armed with that knowledge, we can make smart choices about where to invest our social media, content marketing, PR & media investments, partnerships, and contributions to the web.
In this week’s 5-Minute Whiteboard (which, I apologize, is 9 minutes—the topic demanded a more thorough walkthrough), I’ll explain:
- How LLMs determine which words to put after other words in their responses
- What marketers, founders, and creators can do to influence those results
- Ways to get insight into sources that are likely to be used in an LLM’s training data
- Tactics to find opportunities for influencing that training data
Transcript
How do you get your brand, your company, your name to be the answer that a large language model gives when users ask a relevant question?
This is basically what SEO (search engine optimization) was for Google, Bing, Dogpile, Lycos, and HotBot way back in the day, except these new models, AIs, are doing it completely differently. The currency of Google search was links. The way that you ranked in these results was through links, relevant content, smart keyword use, and references to your work from sources the search engines crawled.
The way that you rank in large language models is not that. The currency of large language models is not links. The currency of large language models is mentions (specifically, words that appear frequently near other words) across the training data. Let me show you what I mean.
I was actually quite embarrassed by these results. I don’t think they’re great. Hence, this video, Google’s AI overview answer is not great either. Even though they do cite Seer Interactive who has some some pretty good stuff in here, and I’ve linked to them in the comments.
Alright. First off, I like, what Stephen Wolfram wrote last year about how ChatGPT works. I think it’s the most clear and accessible answer to how a large language model decides what answer to give. And in his essay about this, “What is ChatGPT doing and why does it work?” he shows this particular graphic, which I think is helpful.
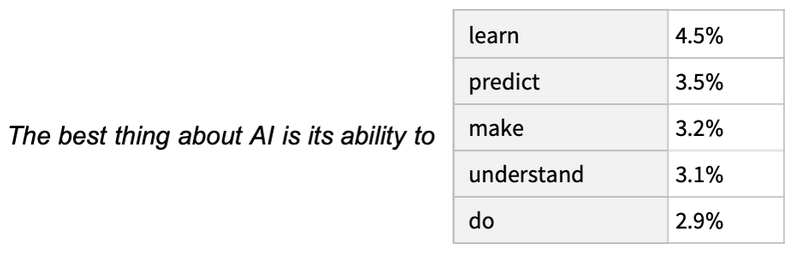
The best thing about AI is its ability to learn, predict, make, understand, and then there’s a percentage.
That percentage, he explains, comes from the training data. Essentially, if ChatGPT has seen that the word “learn” appears much more frequently than the word “predict” when the sentence “the best thing about AI is its ability to…” shows up on the web, they are more likely to show “learn” than “predict.”
That is how you get the large language model output, which some people describe as spicy autocomplete or, words that frequently come after other words. And this is a really good way in my opinion to think about AI tools. So I asked ChatGPT, what are the best fine dining restaurants in Seattle? And, you can see that I’ll I’ll hide this for you.
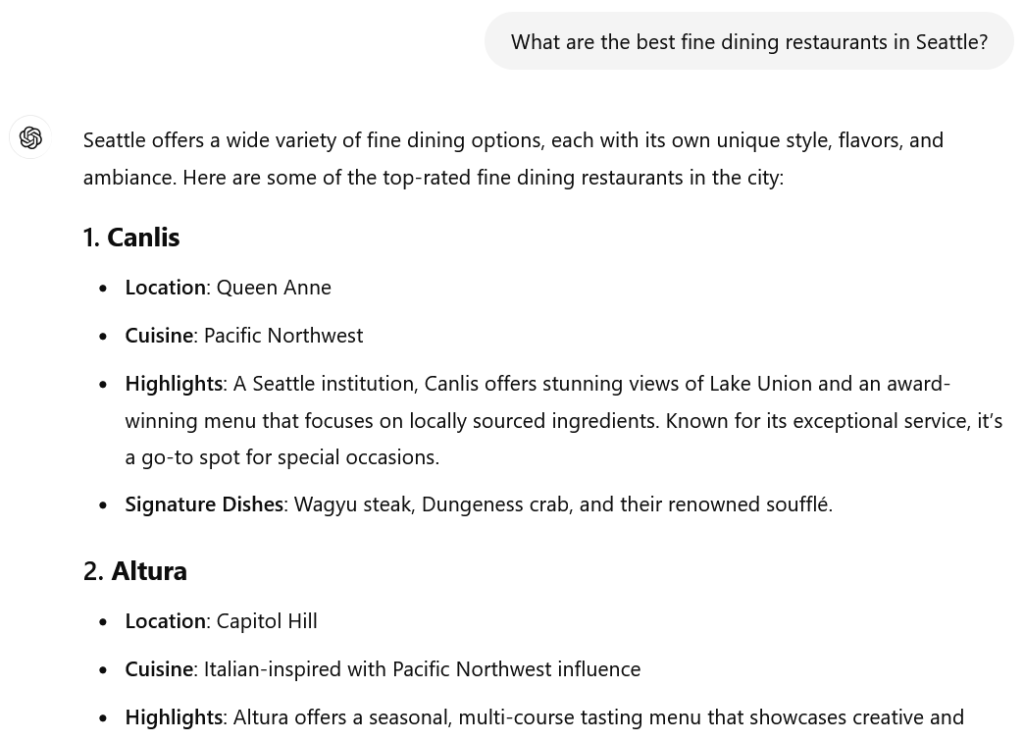
You can see that Canlis is the top result. I actually asked ChatGPT this same question many times. I got different results every time, which is one of the frustrations of a large language model because as Wolfram shows in his example here, it is a percentage chance that you’re gonna see any word that comes after any other word based on the training model or training data. So, Canlis almost always (but not every time) appeared in the first result.
When I ran this, you know, five, ten times, I think I saw it seven out of eight times or something like that. And, these other ones, Herbfarm was frequently in their rovers, which hasn’t existed in many years. And and, unfortunately, they mentioned the chef, Thierry Rautureau, who passed away a year ago. But most of these other ones are reasonable answers, and they’re not that far off of what Google is providing here.
You can see that when I search for fine dining restaurants in Seattle, Canlis is the first result. Altura is the second.
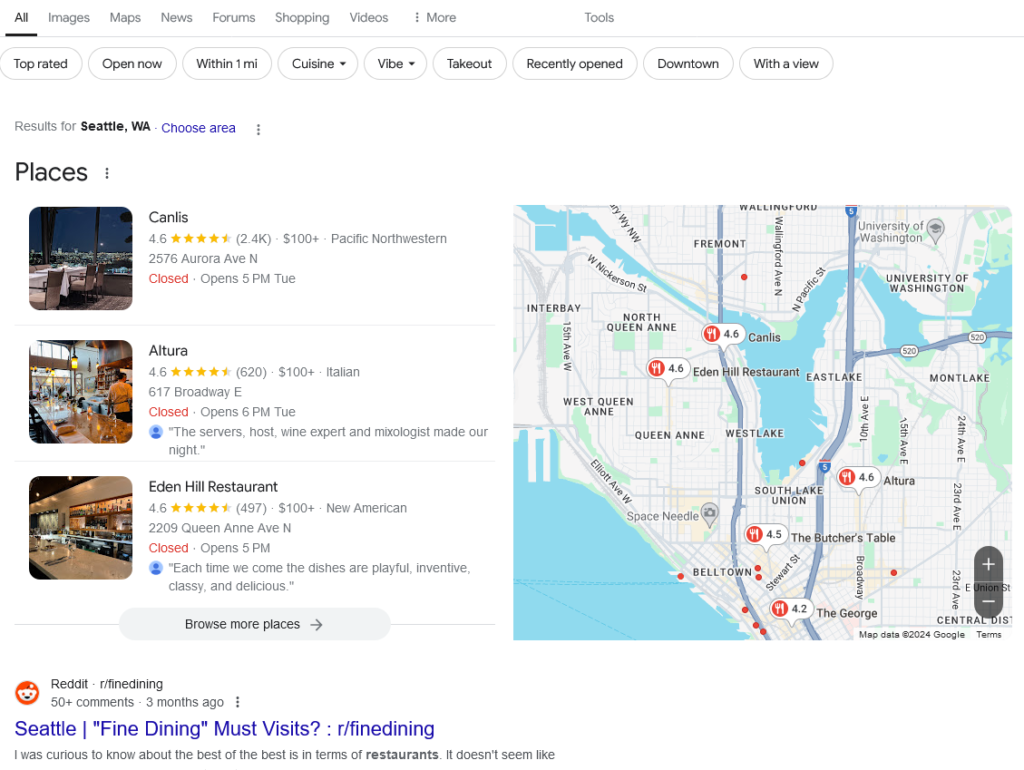
Here is what I would do if you are attempting to get into these results. If you’re, for example, Eden Hill restaurant or one of these other fine dining restaurants in Seattle and you don’t see yourselves mentioned in ChatGPT or other large language model answers, and you think it’s important for your brand or company to appear in these LLM results, what’s the next course of action?
Here is what I would do:
I would look for all the places on the web that talk about, fine dining and restaurants and Seattle. You can see I’ve done this specifically in quotes rather than, trying to put this just as a standard query and that and that’s for a particular reason. That’s so that the results that I see in here use exactly this language because, of course, we are looking for words that frequently come after other words, and so we wanna find those places on the web.
And then what we would like to do is make sure that our brand is mentioned here. Now is it very difficult to get your restaurant mentioned in the best restaurants in Seattle for special occasions list on EATER? Yeah. It is. That’s hard. That’s gonna be a PR process and a pitch process, but it but is it worthwhile?
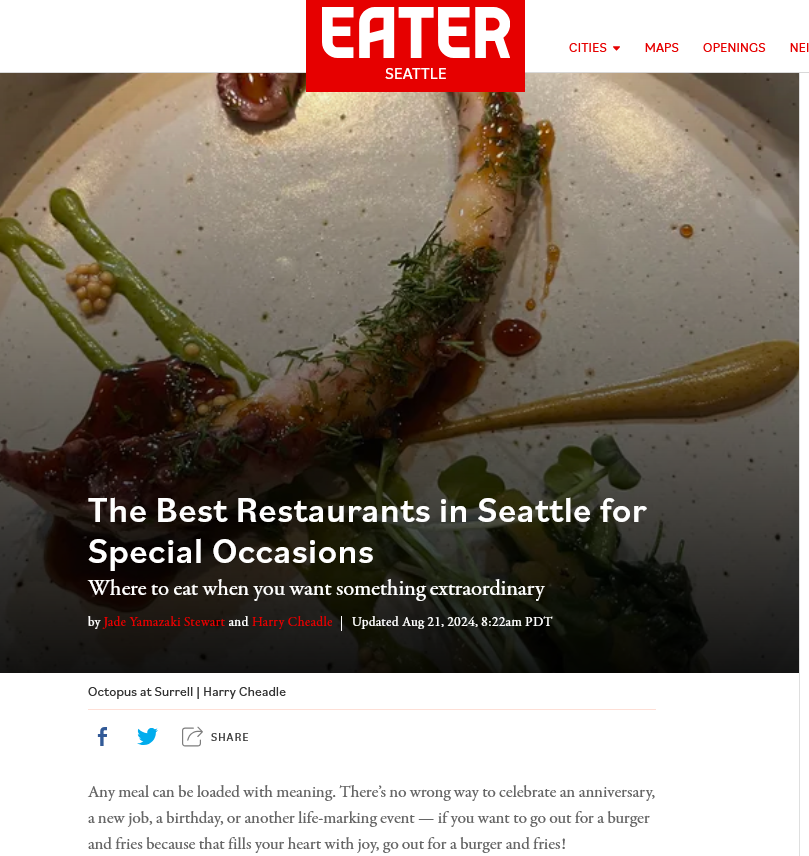
Absolutely. Not only worthwhile for large language models, although certainly, it is likely to be useful for them, but it’s likely that other people will click this result and find you through there. And this is true for almost all of the results that you might find here. So this is a manual process.
It’s one way to go about this. Another way, which is fit fairly interesting, and I actually find that large language models themselves are pretty aware of which websites are likely to contribute to large language model training data. So you can ask ChatGPT or your LLM of choice, you know, what are the odds that Conde Nast Traveler, CN Traveler dot com is likely to be used in LLM training data. And as you can see here, on a scale this is what I asked ChatGPT.
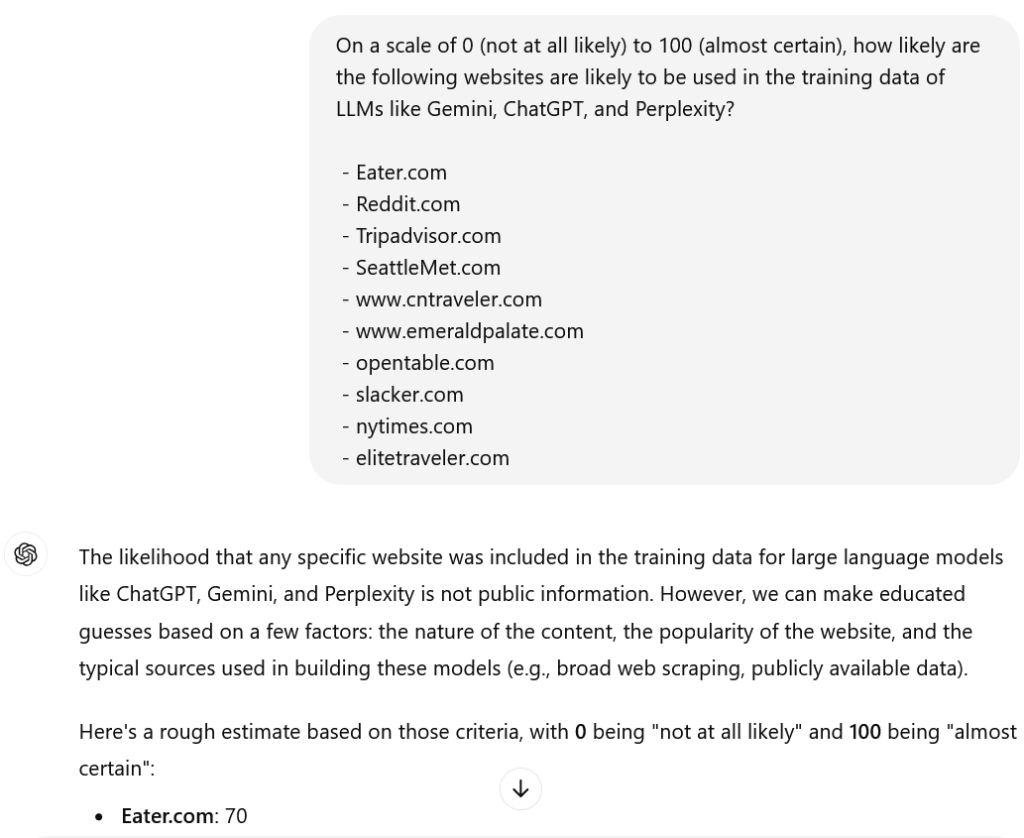
On a scale of zero, not likely at all, to a hundred, almost certain, how likely are the following websites to be used in the training data of large language models like Gemini and ChatGPT and perplexity.
And then I give it a list of sites, and it will give me some estimate. I don’t think these are perfect. If you ask it ten times, again, it’ll give you ten different answers. But it will almost always say things that are true like Reddit is very likely to be used here. We know that they’re selling their data to large language models and so it’s a common source for training data. Eater and CN traveler, and the New York Times, quite high up there. And then smaller sites, more niche sites, places that are harder to crawl, you can see different numbers in there.
And, for example, this user generated content is sometimes excluded for privacy reasons. These are things that are true and have been written about large language model processes, which is really useful. Now let me show you something else that’s pretty slick.
You can go into SparkToro and you can say, hey, for people who search for the keywords fine dining Seattle, what websites are they likely to end up on? What websites are high affinity with this audience? And you can see in here that some of those include things like the Four Seasons, which is a very fancy hotel in Seattle where one might find travelers who are likely to seek out fine dining. You can see things in here like Alaska Air, which is the, Seattle is the hub and, center of Alaska Airlines.
And so no surprise, many visitors to the city come in through there. So this is different from what large language models are likely to use in training. However , you can take this list, export a CSV. You can open up that CSV, and then, copy these domains, and you can do exactly, what I just showed with ChatGPT.
Do it again for this list.
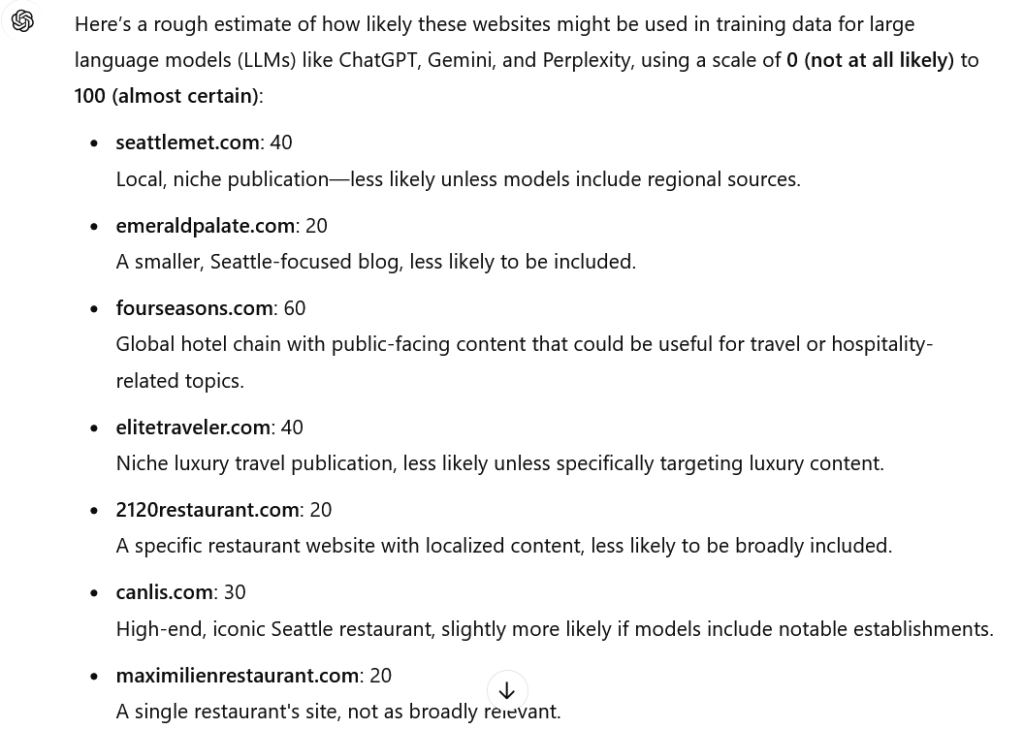
Boom.
And there we are now likely to get, you know, estimates on these types of things. Again, I would not take these results perfectly literally, but I would use them as indicative of where you might need to do PR and comment marketing and, pitches for:
- “Hey, can we write a piece with you?”
- “Can we get into your next edition?”
- “Next time you’re talking about restaurants, we would like our restaurant to be considered.”
- “Can we invite your critic here?”
Whatever the things are that you might do.
And it’s not just SparkToro. There’s another tool I would recommend that you use. This is BuzzSumo. They have an alerting feature that I love and have used for years. So what I’ve done here is create let me just blow this up so you can see it.
So you can create an alert for a keyword. I’m gonna call this my fine dining Seattle, search, and what keyword would I like to monitor? I would like to monitor fine dining with an exact match, match, and it must also contain exactly the keyword Seattle, and I want the digest there. And what BuzzSumo will do that’s nice is it will give me a preview of the results that it’s seen. So where are people on the web publishing content that contain the word fine dining and Seattle. And then you can see these places, right, like Elite Sports Tours and Washington Tasting Room dot com and Eater, which which we know.
This process of essentially doing outreach and PR and content marketing and making sure that your words are appearing on the web after other words in the places where LLMs are likely to source their training data, this is the methodology for how you get your brand into large language model answers.
If, like Canlis, you can become synonymous with fine dining in Seattle, you will appear frequently at the top of results when large language models give them to you.