When site owners and marketers log into their analytics tools to determine how visitors discover and reach their properties, they expect accurate information. Unfortunately, that data is often massively flawed. SparkToro partnered with Really Good Data in a recent experiment to drive 1000+ visits across 11 major social networks and observe how Google Analytics categorized these referrals.
Special thanks to Steve Lamar, an analytics expert who helps folks with GA4 migrations and implementations, for volunteering to set up and run this experiment alongside us.
Results at a Glance
A number of major social networks obfuscate their referred traffic, and a significant, additional set obscure referral data at least some of the time.
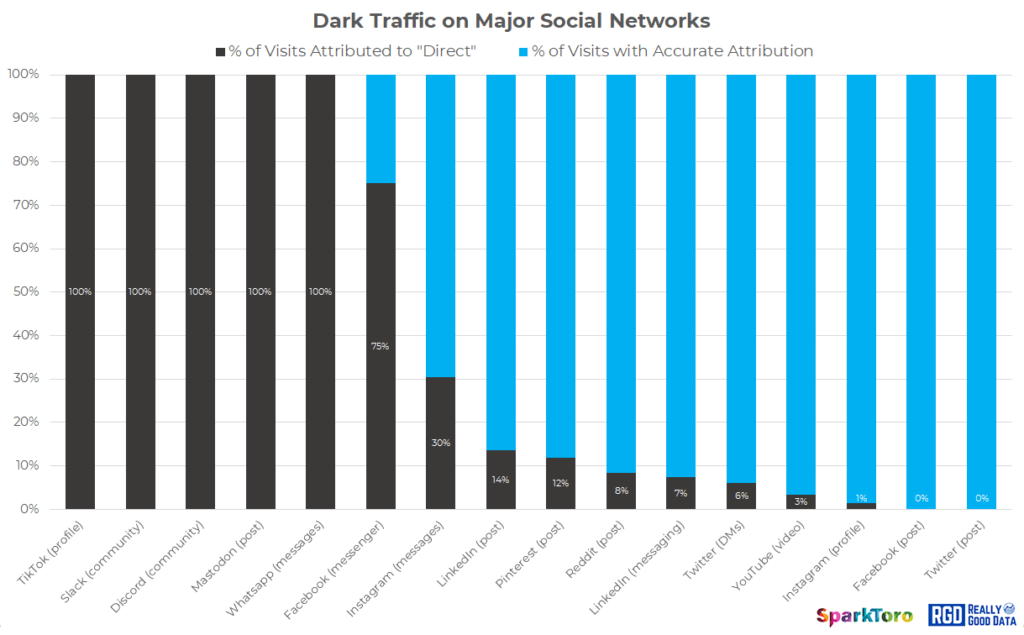
As the chart above shows:
- 100% of all visits from TikTok, Slack, Discord, Mastodon, and WhatsApp were marked as “direct,” and contained no other referral information.
- 75% of visits from Facebook Messenger contain no referral information. This does not appear strictly related to browser choice, device type, or web vs. app.
- Instagram messages (DMs) as well as public LinkedIn and Pinterest posts also missed substantial portions of referral data (30%, 14%, and 12% respectively)
- A smaller amount of traffic was misattributed to “direct” by Reddit posts, LinkedIn messages (DMs), and Twitter DMs
- YouTube, public Instagram profile links, public Facebook posts, and Tweets appear (for now) to provide referral data in most or all cases
In summary, website owners and marketers should expect that a large portion of traffic marked as “direct” in their analytics was likely sent by these networks. Dark Social is a real, ongoing phenomenon with problematic consequences for those who take their analytics’ channel/source reporting at face value.
The Experimentation Process
In order to conduct this research, Steve Lamar (LinkedIn) of Really Good Data set up 16 unique URLs on a subdomain with no other website links, traffic sources, or previous activity. He installed Google Analytics 4 and UA, and built countdown timers on the pages to insure that experiment participants would stay on the pages long enough to trigger a “visit” in the analytics’ systems.
Each page used a unique identifier in the URL to indicate a particular network and traffic type (e.g. /facebook or /facebook-messenger). In this way, as URLs were shared, website analytics could record the visited page, even when referral data was lacking. I.E. A visitor sent the link via Facebook Messenger could only land on /facebook-messenger, and even if that visit lacked any referral data, we were able to know where the click/visit came from.
Rand (Fishkin, of SparkToro) then worked with Steve to create public posts, comments, profile links, communities, subreddits, etc. on each of the networks with links to the associated URLs. We did this somewhat sequentially (only sharing the private DM/messaging URLs via those networks and after the public URL test), which minimized the risk of cross-contamination or spread from one network/referral type to another.
Finally, Rand and Steve then privately recruited a panel of ~100 experiment participants via emails and text messages, asking each to visit the various pages from the associated network/source (e.g. we’d send WhatsApp messages to individuals with the WhatsApp link, emails to the Twitter page containing the URL, etc).
Full Results
Using a simple spreadsheet, we tracked total visits, visits reported as “direct,” and those with correct attribution across each of the pages and networks. Thankfully, we saw no referrals with incorrect attribution (e.g. no one shared or clicked a link to the LinkedIn DMs page from a Twitter or Reddit post), suggesting the integrity of the experiment remained uncompromised throughout the process.
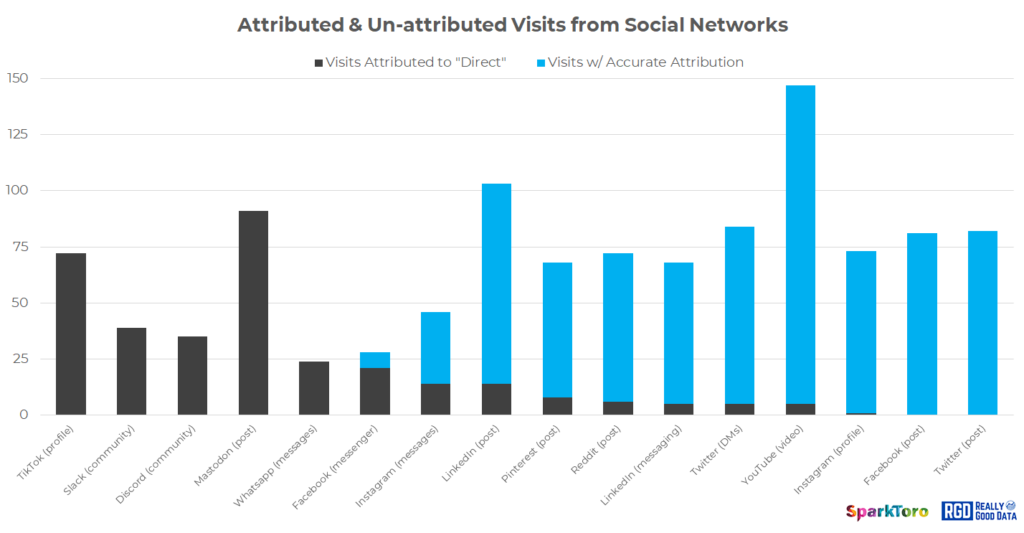
We recorded a grand total of 1,113 visits over 10 days to the 16 unique pages set up across 11 networks (and 5 “private message” systems on those). Below is a full accounting of the unique URLs and networks, and the visits each received from our private panel:
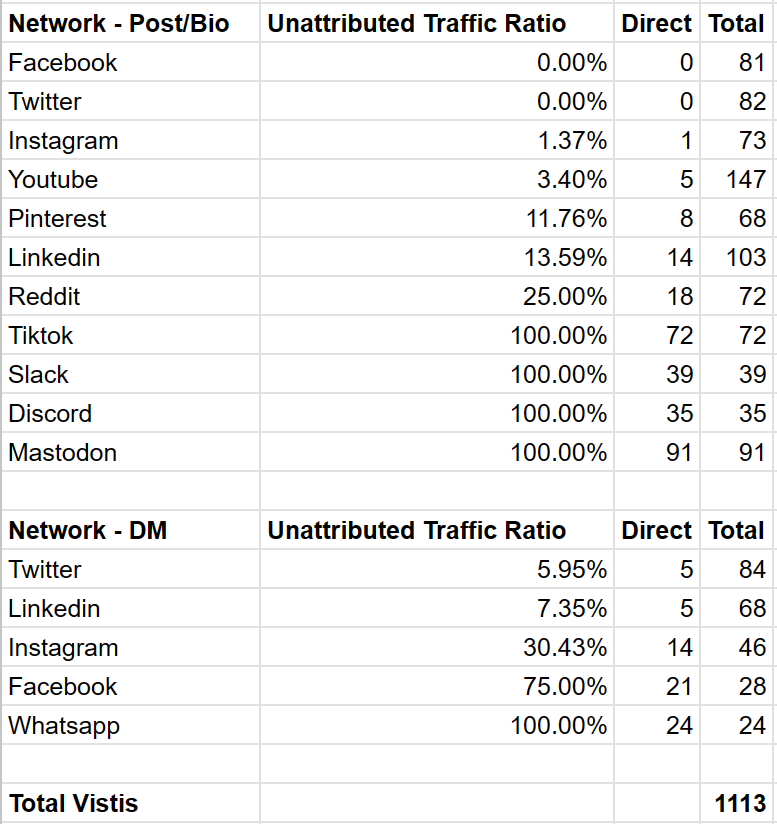
We recorded visits from 6 types of browsers, and a solid breakdown of both mobile and desktop (each participant was asked to use both mobile and desktop, though some only opted to use one).
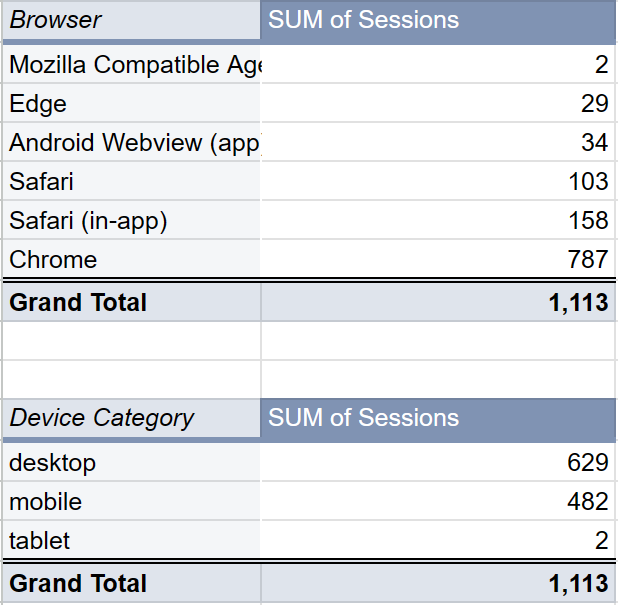
This panel, though substantive, isn’t exhaustive or fully representative of the overall Internet-using population. However, our guess is that, if anything, this group’s behaviors and profiles (primarily US and Canada, only one with ad blockers installed, only one using a privacy-purpose browser, etc.) probably provide more tracking data than a globally-representative worldwide panel would.
Takeaways
If you’re a marketer or website owner reliant on analytics platforms (GA or anything else) to provide accurate, comprehensive information about where your visitors came from and how they found your website, we recommend revisiting those assumptions.
A substantial portion of social referral traffic comes without proper, accurate referral data, and is misclassified as “direct.” If you’re attributing all direct visits to type-in visitors, email, text message, offline/brand campaigns, or Dark Search (the cousin of Dark Social, in which Google, Bing, and other search engines fail to pass referral data of their own), you’re almost certainly undercounting the impact of social media marketing, social referrals, and word-of-mouth on social networks.
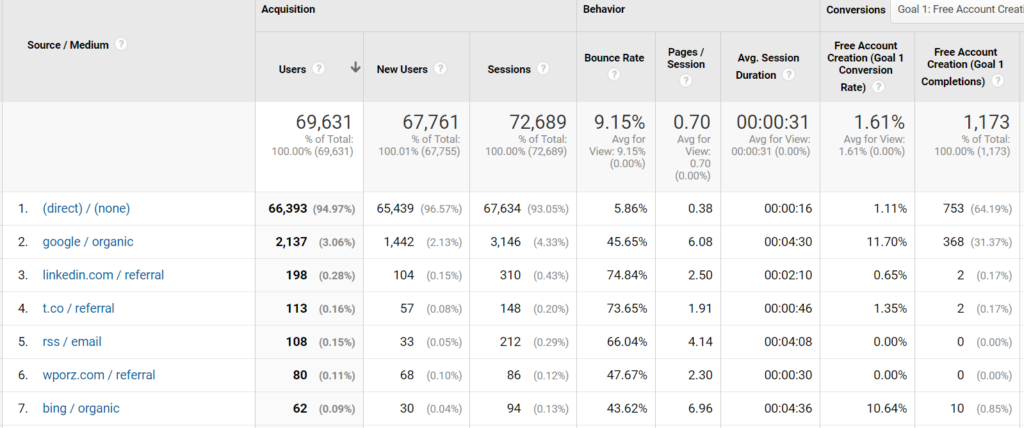
SparkToro gets 95% of its traffic from “Direct” (according to Google Analytics), but we know this isn’t the truth
Even more seriously, if you’re in a sector with heavy use from private communities and messaging groups like Slack, Discord, Facebook Messenger, and WhatsApp, or one whose demographics are likely to use/send traffic via TikTok or Mastodon, you’re probably missing these channels entirely.
For those investing in pro-active social media marketing, we recommend using unique URLs to track activity to social promotions (though you should probably keep them more subtle than the URL format we employed here). But don’t expect this to fully solve the problem. When others share your work, they’re unlikely to be diligent about employing precise social attribution URLs and parameters across the precise networks you’d want. Long story short – the Internet is a messy place, attribution will be wrong sometimes, lost others, and always imperfect.
Next Steps
While this experiment proved successful and informative, we’d love to see more data, from more sources in the future. Our belief is that more widespread sharing (possibly a public version of this experiment) could help expose more of the messy, imperfect reality that operators will face in their online marketing and attribution efforts. If you run something like this, let us know and we can potentially include links to your work here.
In the years ahead, we hope to repeat this experiment as new networks rise in importance and others change their protocols around attribution.