TL;DR – From May 13-15, 2022, SparkToro and Followerwonk conducted a rigorous, joint analysis of five datasets including a variety of active (i.e. tweeting) and non-active accounts. The analysis we believe to be most compelling uses 44,058 public Twitter accounts active in the last 90 days. These accounts were randomly selected, by machine, from a set of 130+ million public, active profiles. Our analysis found that 19.42%, nearly four times Twitter’s Q4 2021 estimate, fit a conservative definition of fake or spam accounts (i.e. our analysis likely undercounts). Details and methodology are provided in the full report below.
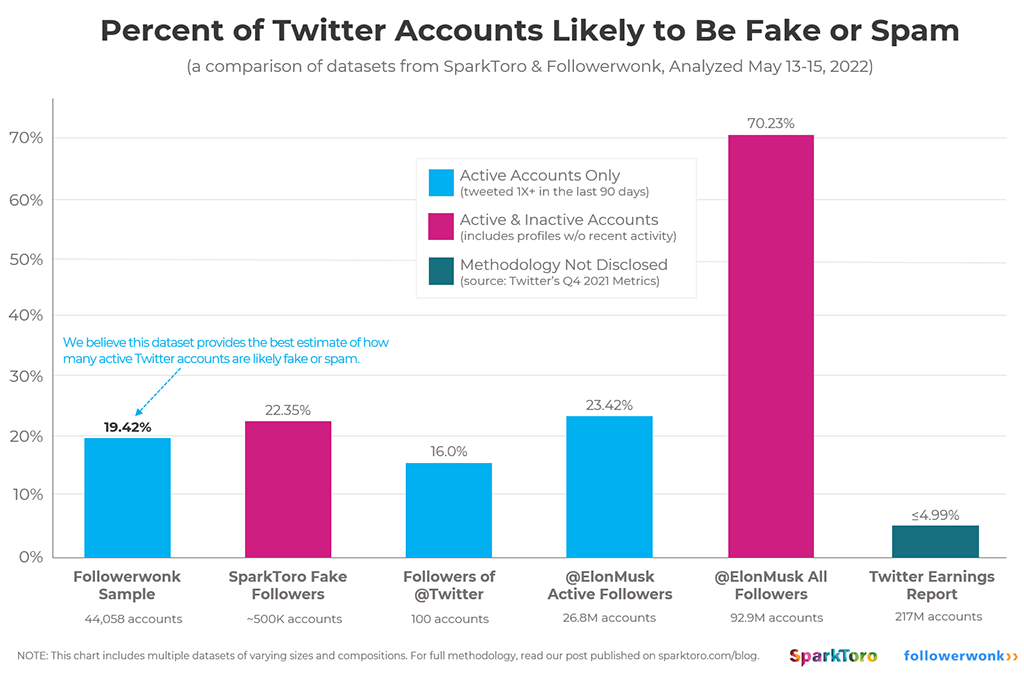
For the past three years, SparkToro has operated a free tool for Twitter profiles called Fake Followers. Over the last month, numerous media outlets and other curious parties have used the tool to analyze would-be-Twitter-buyer, Elon Musk’s, followers. On Friday, Mr. Musk tweeted that his acquisition of Twitter was “on hold” due to questions about what percent of Twitter’s users are spam or fake accounts.
Mr. Musk’s tweet from Friday, May 13
SparkToro is a tiny team of just three, and Fake Followers is intended for informal, free research (our actual business is audience research software). However, in light of significant public interest, we joined forces with Twitter research tool, Followerwonk (whose owner, Marc Mims, is a longtime friend) to conduct a rigorous analysis answering:
- What is a spam or fake Twitter account?
- What percent of active Twitter accounts are spam or fake?
- What percent of Mr. Musk’s followers are spam, fake, or inactive?
- Why should our methodology be trusted?
We address each of these questions below.
What is a Spam or Fake Twitter Account?
Our definition (which may differ from Twitter’s own) can best be described as follows:
“Spam or Fake Twitter accounts are those that do not regularly have a human being personally composing the content of their tweets, consuming the activity on their timeline, or engaging in the Twitter ecosystem.”
Many “fake” accounts under this definition are neither nefarious nor problematic. For example, quite a few users find value in following a bot like @newsycombinator (which automatically shares frontpage posts from the Hacker News website) or @_restaurant_bot (which tweets photos and links from random restaurants discovered through Google Maps). These accounts, arguably, make Twitter a better place. They just don’t have a human being behind a device, personally engaging with the Twitter ecosystem.
By contrast, most “spam” accounts are an unwanted nuisance. Their activities range from peddling propaganda and disinformation to those attempting to sell products, induce website clicks, push phishing attempts or malware, manipulate stocks or cryptocurrencies, and (perhaps worst) harass or intimidate users of the platform.
SparkToro’s Fake Followers methodology (described in detail below) attempts to identify all of these types of inauthentic users.
Our systems do not, however, attempt to identify Twitter accounts that may be irregularly operated by a human but have some automated behaviors (e.g. a company account with multiple users, like our own @SparkToro, or a community account run by a single person, like Aleyda Solis’ @CrawlingMondays). We cannot know how Twitter (or Mr. Musk) might choose to classify these accounts, but we bias to a relatively conservative interpretation of “Spam/Fake.”
What Percent of Active Twitter Accounts are Spam or Fake?
To get the most comprehensive possible answer, we applied a single spam/fake account analysis process (described below) across five unique datasets. These are visualized in the graph below (the same one that appears at the top of this post).
The datasets represented above are:
- Followerwonk Random Sample (44,058 accounts) – Followerwonk currently has 1.047 Billion Twitter profiles indexed, updated in a continuous cycle that takes ~30 days. Any account that has been deleted (by the user or Twitter) gets removed and is not included in the count. Of those, 130 Million are “recently active” by Followerwonk’s definition, i.e. they’ve sent tweets within the past 9 weeks, and are public, not “protected” (Twitter’s terminology for private accounts).
Marc wrote code to randomly select public accounts from Followerwonk’s active database, and passed them to SparkToro for analysis. Casey on our team further scrubbed this list and ran 44,058 public, active accounts through our Fake Followers spam analysis process, finding 8,555 to have an overlap of features highly correlated with fake/spam accounts. We believe this dataset represents the best, single answer to the question of how many active Twitter users are likely to be spam or fake. - Aggregated Average of the Fake Followers Tool (~500K profiles run, 1B+ accounts analyzed) – Over the last 3.5 years of operation, SparkToro’s Fake Followers tool has been run on 501,532 unique accounts, and analyzed thousands of followers for each of those, totaling more than 1 billion profiles (though these are not necessarily unique, and we don’t keep track of which profiles were analyzed as part of that process).
This represents the largest set of accounts on Twitter we could acquire, but it includes analysis of many older accounts that haven’t sent tweets in the last 90 days and thus, likely don’t fit Twitter’s definition of mDAUs (monetizable Daily Active Users). We’ve included it for comparison, and to show that an analysis that includes simply random Twitter accounts (vs. those that have been recently active) may not be as accurate. - All Followers of @ElonMusk on Twitter (93.4M accounts) – Given the unique interest in Mr. Musk’s account, and the central role it played in triggering this report, we felt it wise to include a complete analysis of the nearly hundred million accounts that follow @ElonMusk. This dataset includes older profiles that haven’t tweeted in the last 90 days (and don’t fit Twitter’s mDAUs definition).
- Active Followers of @ElonMusk on Twitter (26.8M accounts) – A more fair assessment of Mr. Musk’s Twitter following would only include accounts that have tweeted in the past 90 days. In order to match the methodology used in our Followerwonk analysis, we selected only those 26,878,729 matching this criteria and have broken them out in the chart above.
- Random Sample of 100 Users Following the @Twitter account (100 accounts) – In a follow-up to his tweet on Friday, May 13th, Mr. Musk said that “my team will do a random sample of 100 followers of @twitter; I invite others to repeat the same process and see what they discover.”
While we don’t believe this process to be a rigorous, statistically significant sample set, we’ve nevertheless included it for comparison purposes. On Saturday, May 14th, we manually took a random sample of accounts from the public page of @Twitter’s followers here. In order to get the least biased sample, we included only public accounts, only those that sent tweets in the past 90 days (after Feb 12th, 2022), and only accounts created before May, 2021, i.e. they’ve been on Twitter 1+ years (many recent accounts, especially in light of Mr. Musk’s activities, could bias the sample). - Twitter’s Most Recent Earnings Report Estimate (Unknown number of accounts) – Twitter’s public earnings report, quoted by Mr. Musk in his recent tweet, shares that <5% of mDAUs (monetizable Daily Active Users, defined in their 2019 report here) are fake or spam. We’ve included this estimate in the chart for comparison, and noted that the methodology is undisclosed.
Undoubtedly, other estimates will be made by other researchers, hopefully with equally large and rigorous datasets. Given the limitations of publicly available data from Twitter, we believe the most accurate estimate to be: 19.42% of public accounts that sent a tweet in the past 90 days are fake or spam.
What Percent of Elon Musk’s Twitter Followers are Spam, Fake, or Inactive?
In October of 2018, SparkToro analyzed all 54,788,369 of then US President, Donald Trump’s, followers on Twitter. We replicated that process for this report, analyzing all of Elon Musk’s profile’s 93,452,093 followers (as of May 14, 2022).
When running a Fake Followers report through our public tool, we analyze a sample (several thousand) of a Twitter user’s followers. When an account has a very large number of followers, this methodology can deviate from what a full analysis of every follower shows. Over Saturday, May 14th and Sunday, May 15th, SparkToro’s Casey Henry spun up this comprehensive analysis for Mr. Musk’s account, to provide the most precise number possible.
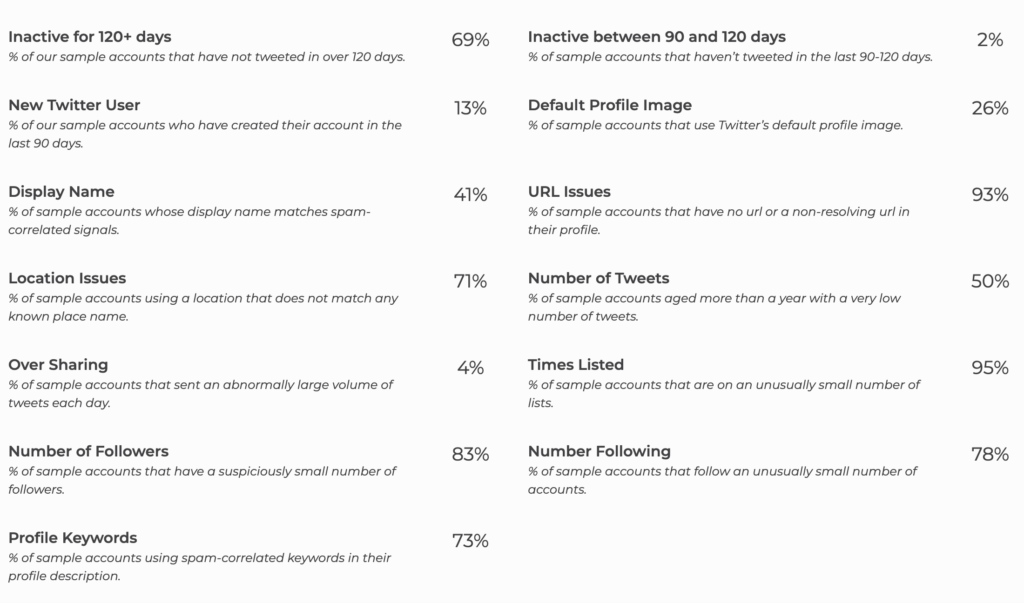
The breakdown of some factors used in our spam analysis system is above, and in total, 70.23% of @ElonMusk followers are unlikely to be authentic, active users who see his tweets. This is well above the median for fake followers, but is unsurprising (to us, at least) for several reasons:
- Very large accounts tend to have more fake/spam followers than others
- Accounts that receive great deals of press coverage and public interest (like ex-President Trump and Mr. Musk) tend to attract more fake/spam followers than others
- Accounts that Twitter recommends to new users (which often includes @ElonMusk) tend to get more fake/spam followers
When compared to the distributions of other Twitter accounts, @ElonMusk’s fake/spam follower count may seem out of the ordinary, but we do not believe or suggest that Mr. Musk is directly responsible for acquiring these suspicious followers. The most likely explanation is a combination of the factors above, exacerbated by Mr. Musk’s active use of Twitter, the media coverage of his tweets, and Twitter’s own recommendation systems.
We also conducted an analysis of only those 26.8M @ElonMusk followers who’ve tweeted in the last 90 days. This filter matches the one we applied to the Followerwonk dataset and the random followers of @Twitter.
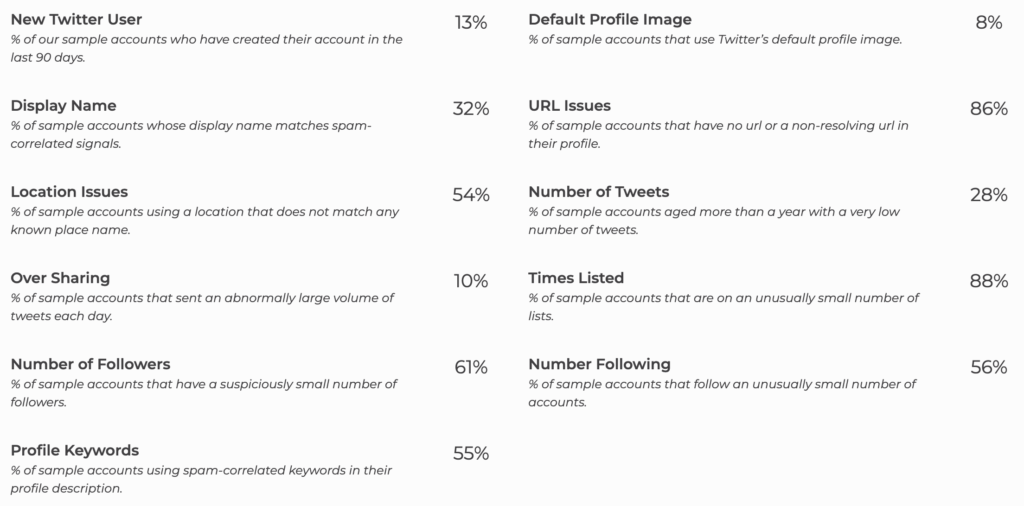
This more selective analysis found 23.42% to be likely fake or spam, a number not far off the estimated, global average.
Why Should SparkToro & Followerwonk’s Methodology Be Trusted?
The datasets analyzed above (save for the random 100 followers of @Twitter, a methodology we don’t endorse) are large enough in scope and rigorous enough in process that their results are reproduceable by any Twitter researcher with similar public access. We invite anyone interested to replicate the process we’ve used here (and describe in more detail below) on their own datasets. Twitter provides information on their API offerings here.
Followerwonk selected a random sample from only those accounts that had public tweets published to their profile in the last 90 days, a clear indication of “activity.” Further, Followerwonk regularly updates its profile database (every 30 days) to remove any protected or deleted accounts. We believe this sample is both large enough in size to be statistically significant, and curated to most closely resemble what Twitter might consider a monetizable Daily Active User (mDAU).
SparkToro’s Fake Followers analysis consider an account fake if it triggers numerous signals SparkToro shows in our Fake Followers tool:
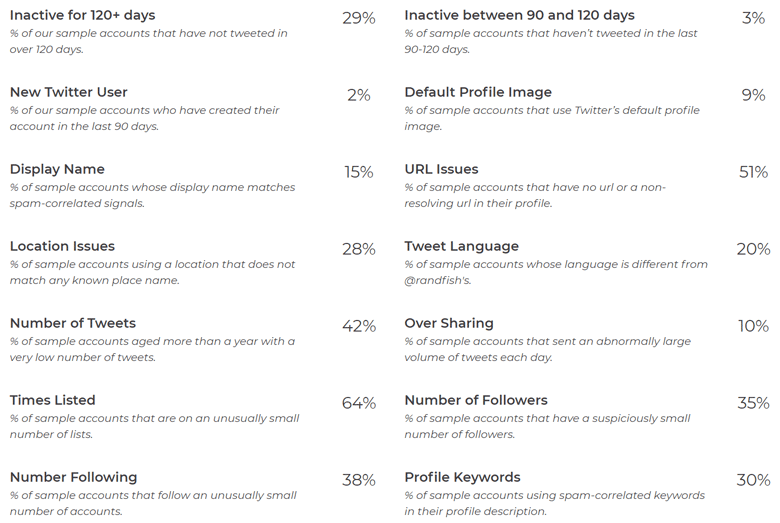
Above: some of the 17 signals SparkToro’s system uses to identify fake or spam accounts.
Our model for identifying fake accounts comes from a machine learning process run over many tens of thousands of known spam (and real) Twitter accounts. Here’s how we built that model:
In July of 2018, we bought 35,000 fake Twitter followers from 3 different vendors of spam and bot accounts. Our vendors had those accounts follow an empty Twitter account, created in 2016, that had 0 followers in July 2018. It took ~3 weeks to deliver the 35,000 followers. Every day for the next 3 weeks, we collected data on these fake/spam accounts.
In addition to those 35,000 known spam accounts, we took another random sample of 50,000 non-spam accounts from a SparkToro’s large index of profiles. This gave us a total of 85,000 accounts to run through a machine learning process on Amazon Web Services.
Those 85,000 accounts were split into two groups with a mix of SPAM and non-SPAM accounts. Group A as the training set, and Group B as the testing set to analyze performance of the models.
The following data was used for the initial model generation:
- Profile image
- Profile URL
- Verified account status
- Language
- Tweet language
- Account age in days
- Length of bio
- Number of followers
- Number of account they follow
- Days since last tweet
- Number of tweets
- Number of times the account appears on lists
- Location
- Display name
After a model was found to fit the data, we analyzed the results to determine features that closely correlate to spam. Unsurprisingly, no single feature was 1:1 correlated with spam. But, a good number of features showed promise when used in combination. The following are examples of features that correlate to spam accounts:
- Profile image – accounts lacking these are often spam
- Account age in days – certain patterns are clearly spam-correlated (e.g. when a large number of accounts created on a single day follow particular accounts or send nearly identical tweets)
- Number of followers – spam accounts tend to have very few followers
- Days since last tweet – many spam accounts rarely send tweets and do so in coordinated fashions
- Number of times the account appears on lists – spam accounts are almost never on lists
- Display name – certain keywords and patterns correlate strongly with spam
These, however, are not alone, and other signals that have decent correlation with spam (especially when multiple signals apply to a single account) were also useful to build a functioning model. Through trial and error (and, of course, pattern-fitting) we crafted a scoring system that could correctly identify over 65% of the spam accounts. We intentionally biased to missing some fake/spam accounts rather than accidentally marking any real accounts incorrectly.
It’s crucial to keep in mind that no one factor tells us that an account is spam! The more spam signals triggered, the more likely an account is to be spam. Our Fake Followers system requires that at least a handful and sometimes as many as 10+ of the 17 spam signals be present (depending on which signals, and how predictive they are) before grading an account as “low quality,” or fake.
This methodology likely undercounts spam and fake accounts, but almost never includes false positives (i.e. claiming an account is fake when it isn’t).
Applying this model to the ~44K random, recently-active accounts provided by Followerwonk produces a quality score for each account, visualized below:
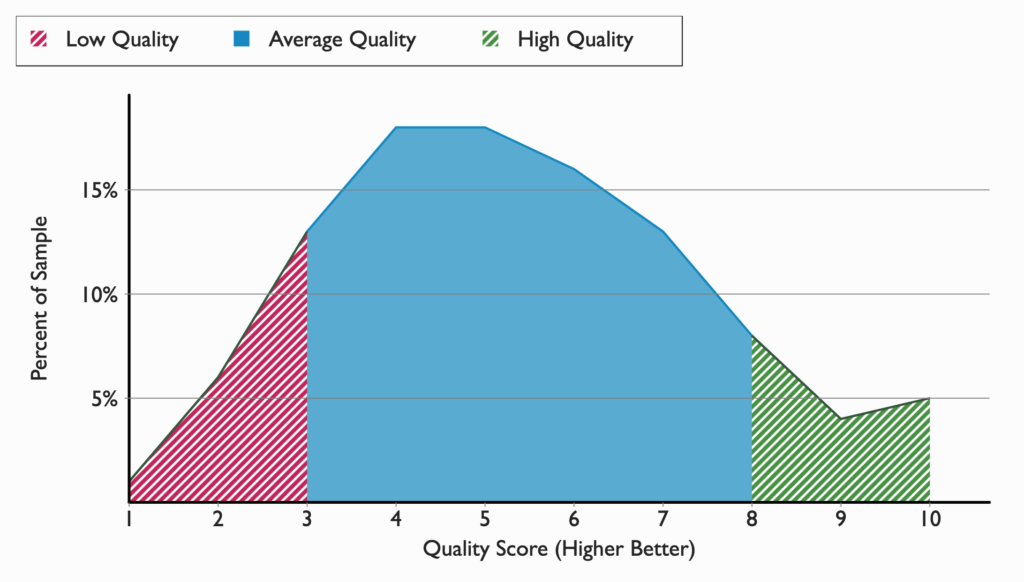
Above: quality score distribution of the 44,058 Twitter accounts analyzed in the Followerwonk Sample Set
The more spam-correlated flags an account triggers, the lower its Quality Score in this system will be. Our conservative approach means that we only treat scores of 3, 2, and 1 as fake/spam accounts, and it’s the combination of these three buckets that produces our final estimate, best-stated as: 19.42% of recently active, public Twitter profiles are extremely likely to be fake or spam.
Before concluding this post, I will pre-emptively address some potential questions:
- Are you challenging Twitter’s earnings report, saying that <5% of mDAUs are fake/spam?
- We are not disputing Twitter’s claim. There’s no way to know what criteria Twitter uses to identify a “monetizable daily active user” (mDAU) nor how they classify “fake/spam” accounts. We believe our methodology (detailed above) to be the best system available to public researchers. But, internally, Twitter likely has unknowable processes that we cannot replicate with only their public data.
- Does this data provide Mr. Musk with reason to break his acquisition agreement with Twitter?
- The four of us who worked on this research are not attorneys, nor are we privy to the specifics of the discussions between Twitter and Mr. Musk. We won’t try to speculate on whether this data will have any impact beyond satisfying collective curiosities.
- What are the most significant flaws, holes, or critiques of the methodology used in this report that could make it inaccurate?
- The most salient critique is that our methodology to calculate an active Twitter user is less accurate than Twitter’s own system. We do not know if an account logged in to view their timeline, or visited Twitter’s website, only if that account sent a public tweet. We undercount active users whose accounts are protected, accounts that view tweets but don’t send any, and accounts that log in and engage in other ways beyond tweeting (like favoriting or adding profiles to lists).
- The other potential critique is our spam/fake follower calculation methodology. Because we crafted it in 2018, based off sample sets of purchased spam accounts, it’s likely that more sophisticated spammers and fake accounts go unidentified by our system. We also bias to a very conservative measure of spam, intentionally missing many likely spam/fake accounts in order not to accidentally mark real accounts as fake. It’s probable that our numbers are lower than more sophisticated, more recently built spam analysis models would show.
- Would larger analyses have made a difference?
- Given the sample size we used (44K / 150 million), we can say with 99% confidence that the spam/fake conclusion of 19.42% is accurate to within ±0.486% on the Followerwonk dataset. Increasing the sample size to 100K only reduces the margin of error to ±0.322%. Where there are inaccuracies in the study, it’s unlikely to be because of sample size.
- Does Twitter’s CEO’s statement that Twitter removes 500k spam/bot accounts per day have an effect on these analyses?
- From Mr. Agrawal’s statement, we can see that Twitter does most of its spam filtering on very new accounts. And this is replicable through the API: it’s difficult to discover very new accounts (though Followerwonk does attempt to do that through various methods). So, many of the spam accounts Twitter removes would never live long enough to make it into our sample. That means the estimate of 19.42% is low. That’s another point where the analysis is conservative.
- FWIW, the average account age in the Followerwonk sample is just under 5 years, Which in turn means the average, active, public account on Twitter is just under 5 years old. The median age is 3.7 years. That implies the spam/fake accounts your model identified have survived Twitter’s scrutiny for a very long time.
- By excluding accounts that don’t tweet or reply in the Followerwonk sample, are you overcounting spam in Twitter’s overall ecosystem?
- It is possible, but for a variety of reasons, we find this unlikely.
- First: Accounts that tweet have far more signals that can be analyzed vs. those that never do. Accounts that tweet are also much more likely to bother real users and get reported. If Twitter were to take action, it seems most salient and important to take them on accounts that cause problems (i.e. tweet or reply) vs. those that don’t.
- Second: In the two large analyses (of Elon Musk’s 93M followers and of the historic 500K Fake Followers profiles), the counts of spam/fake accounts is quite a bit higher than in the analysis of only those that have actively tweeted recently. Including non-tweeting accounts in the Followerwonk sample would almost certainly have returned higher, not lower spam/fake percentages.
- Third: The most easily purchaseable spam and fake accounts are those used to inflate follower counts. As of this writing, they are readily available in vast quantities from dozens of easy-to-find online purveyors. From our experience, these follower-count-inflating accounts last for years, and sometimes indefinitely. Most Twitter users will note that it’s exceptionally rare for follower counts to go down, especially in large quantities. This discrepancy is challenging to square with the idea that Twitter is better at removing fake accounts that never tweet than those who do.
- Can SparkToro or Followerwonk run custom analyses of specific Twitter data or accounts?
- SparkToro does not offer services like this beyond the free Fake Followers tool (and, after a busy few days on this project, our team needs to focus on our core business 😉).
- Followerwonk, however, offers a robust set of Twitter analysis data in its public tool, and may be able to complete specific requests so long as they are in accordance with Twitter’s terms of use. Drop a line to [email protected] and he may be able to assist.
- How should media or other interested parties get in touch?
- If you’re seeking a quote or have other questions for the authors of this research (i.e. Casey, Amanda, Rand, & Marc), drop a line to [email protected]
Thanks to everyone who’s helped to spur and support this research, and especially to Casey Henry and Marc Mims, whose rapid and tireless weekend work made this report possible. As massive fans of Twitter’s platform, and the kindness shown by so many folks there, we’re honored to (hopefully) contribute to its continuing improvements.